What are Artificial Intelligence-Designed Drugs?
Artificial intelligence (AI) is increasingly being used to aid in the discovery and development of new drugs. AI-designed drugs are medications that leverage machine learning algorithms and enormous datasets at some point during the research and development process. Rather than scientists manually screening millions of chemical compounds, AI systems can quickly analyze vast genetic and protein data to identify promising drug targets and design molecules to interact with those targets.
The primary advantage of using AI for drug discovery is the potential to dramatically reduce the time and costs involved. Traditionally, getting a new drug from initial discovery through clinical trials and regulatory approval is an excruciatingly slow process that can take over a decade and cost billions of dollars. By streamlining candidate identification and molecule design, AI could make the entire pipeline significantly more efficient.
Milestones of Artificial Intelligence Designed Drugs
Far from a futuristic concept, AI-enabled drug discovery is already underway and achieving notable milestones.
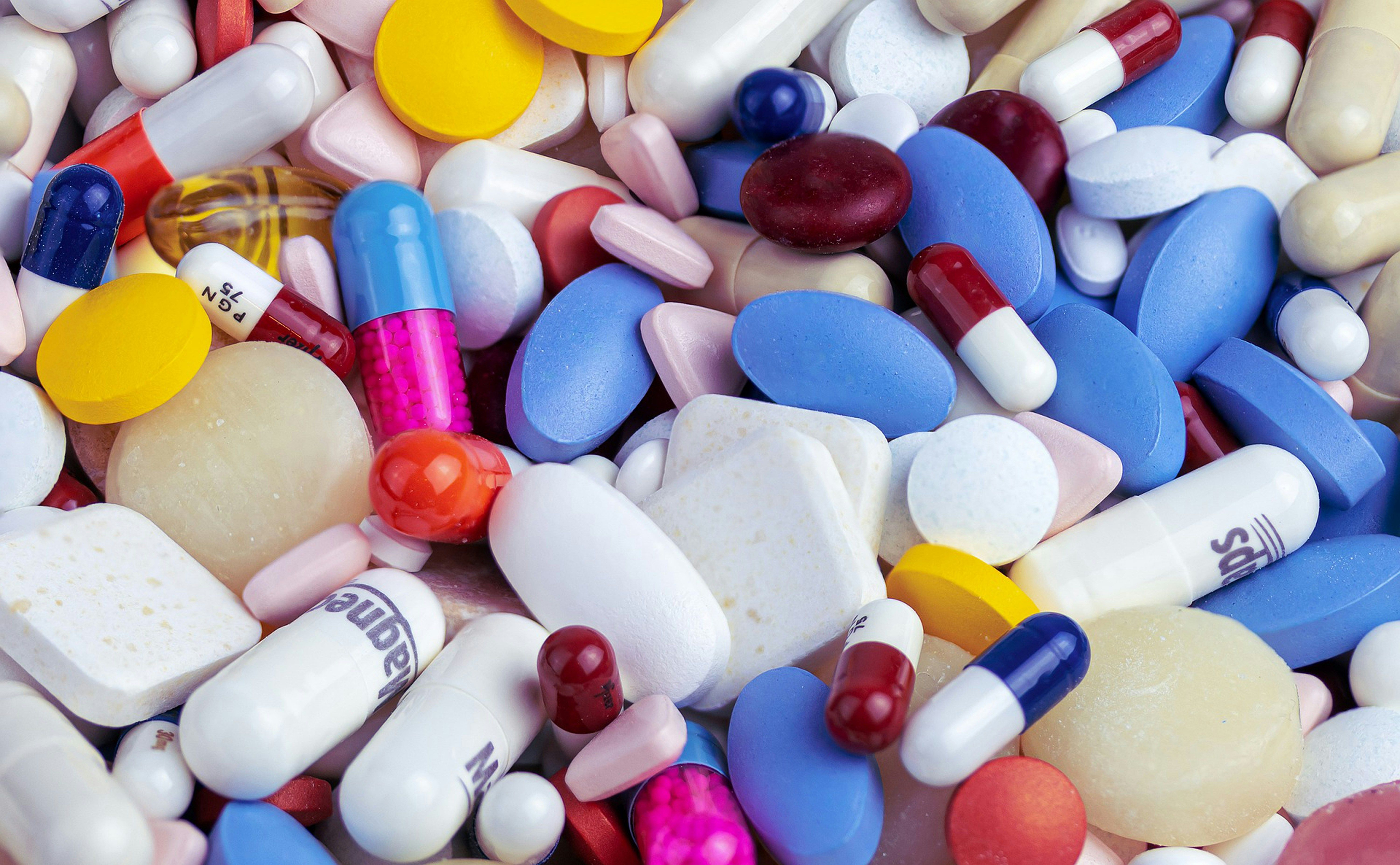
Photo from: Unsplash
- In early 2020, Exscientia announced the first-ever AI-designed drug molecule to enter human clinical trials.
- In 2021, DeepMind's AlphaFold system predicted the 3D structures for hundreds of thousands of proteins, including the entire human proteome. This structural database is an invaluable tool for targeted drug design.
- In 2022, Insilico Medicine initiated Phase 1 trials for what they claim is the first AI-discovered drug candidate targeting a novel AI-identified protein - all achieved at a fraction of the typical time and expense.
- Just this year, AbSci used generative AI to create and validate completely new antibody structures, illustrating AI's ability to go beyond optimizing known molecules.
Current Ongoings in AI and Pharma
The pharmaceutical industry is taking AI-driven drug discovery seriously, with major investments and initiatives.
This week, venture capital firms announced the formation and $1 billion funding of Xaira Therapeutics, a new AI biotech company led by former Genentech executive Marc Tessier-Lavigne. Backed by an impressive syndicate of investors, Xaira intends to develop "drugs that were impossible to make without recent breakthroughs in AI."
Beyond well-funded startups, established players are also deploying AI across all stages of drug research and development. Generative models can craft novel molecular structures optimized for desired properties. AI systems like AlphaFold, ESMFold, and RoseTTAFold provide high-accuracy protein structure predictions to guide targeted drug design.
While representing a major milestone, as of mid-2023 no clinical programs have yet advanced from candidates first discovered by systems like AlphaFold. And despite tremendous progress, no AI-designed drug has received full FDA approval for market entry.
FDA-Approved AI-Designed Drugs?
Although none have reached pharmacy shelves yet, some promising AI-driven drug candidates have cleared initial regulatory hurdles.
DSP-1181 - In 2020, the first-ever AI-designed drug molecule entered clinical trials. Developed by Exscientia and Sumitomo Dainippon Pharma to treat obsessive-compulsive disorder, DSP-1181 went from initial discovery to human testing in just 12 months thanks to AI-accelerated design (compared to 5 years for typical drug candidates). However, it did not advance past Phase 1 trials after failing to meet study goals.
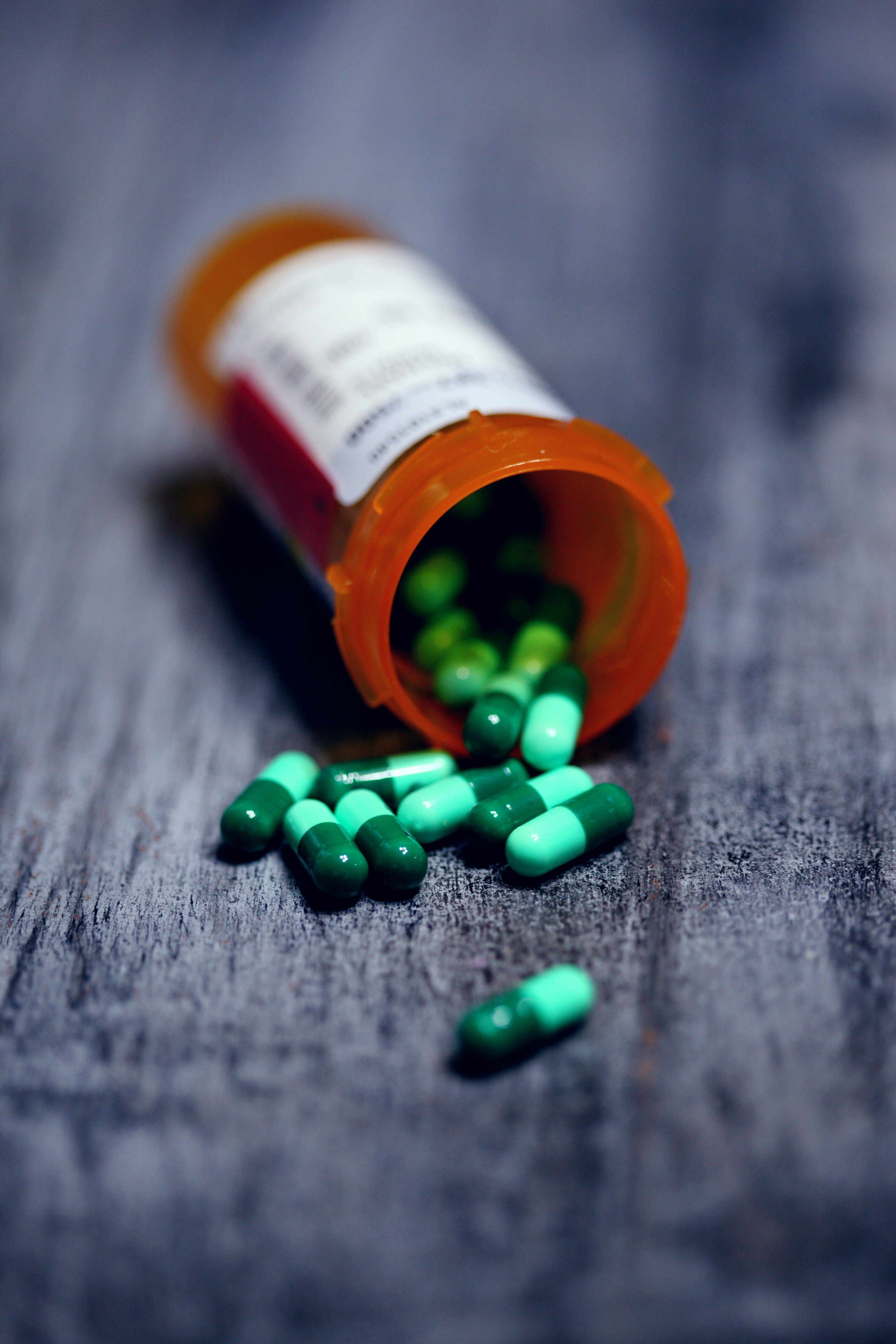
Photo from: Unsplash
INS018-055 - Created by Insilico Medicine, this AI-discovered and designed anti-fibrotic compound is the first to have both its protein target and molecule structure determined using AI from the start. After completing preclinical testing, it entered Phase 1 human trials in 2022 (NCT05154240 study).
Challenges and Future Opportunities for AI Pharmaceuticals
While the future of AI-augmented pharmaceuticals appears promising, several challenges need to be addressed:
Data Limitations - Training effective AI models require massive datasets, which can be lacking especially for rare diseases. Without sufficient data, it's difficult to validate AI-designed drugs for safety and efficacy to satisfy regulatory requirements.
Interpretability Issues - For complex AI systems, understanding the precise mechanisms by which they operate and identify targets/molecules may be opaque, creating challenges in predicting drug behaviors.
Regulatory Hurdles - The FDA approval process is conservative by design to protect patient safety. Ensuring compliance for novel AI-driven drug discovery methods and manufacturing processes will require rigorous validation.
With their superior speed and capacity compared to traditional techniques, AI systems could democratize drug development by lowering entry barriers for more researchers, institutions, and commercial entities to pursue therapeutic candidates. However, there are also potential negative implications to consider.
The Potential Flip Side of Artificial Intelligence Drugs
While most AI drug development focuses on treatments for disease, the same powerful technology could theoretically be turned towards developing recreational drugs, chemical weapons, or other dangerous substances if proper safeguards aren't implemented. Just as AI democratizes therapeutic research, it lowers barriers for bad actors as well.
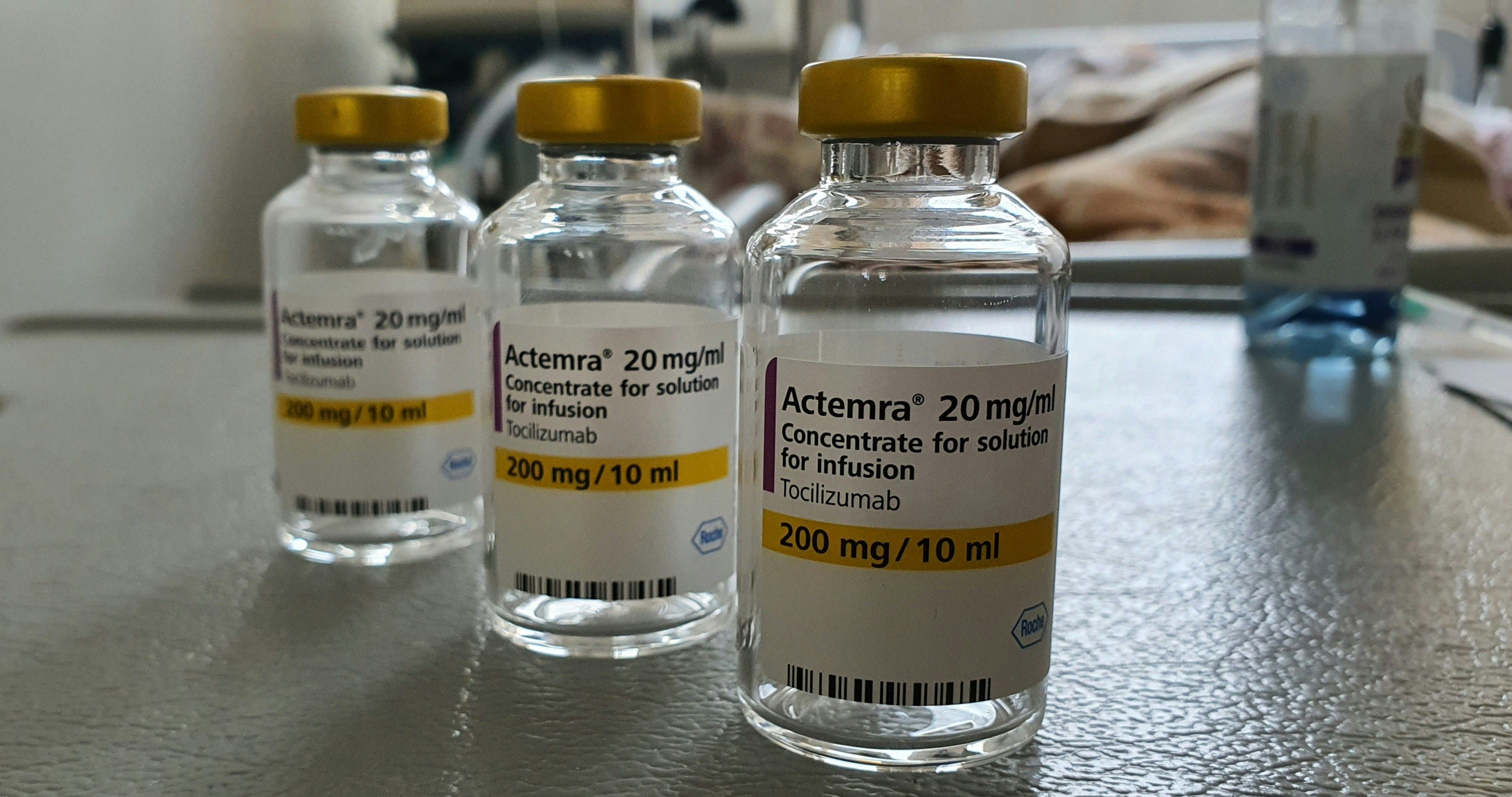
Photo from: Unsplash
The efficiencies of AI-driven molecular design processes could make the illicit manufacture of drugs like opioids, stimulants, or psychedelics far easier and cheaper. AI-optimized versions could be more potent, resist drug testing, or evade law enforcement detection. Criminal organizations already take advantage of innovations like dark web markets and crypto-currencies - the widespread availability of powerful generative chemistry AI models poses a new risk to be mitigated.
Beyond recreational drugs, the same technologies could potentially aid in the design of chemical weapons or other regulated materials. AI structure prediction could theoretically be applied to optimize the toxic properties of nerve agents, choking agents, or banned chemical munitions. While rare talent and access to hazardous precursors impose practical limitations, AI lowers many barriers.
Perhaps most concerning are the potential mental health implications if drug development becomes too efficient and ubiquitous. The widespread availability of mood-altering, dependency-inducing substances could increase rates of addiction and recreational abuse. If AI-designed drugs become very inexpensive and circumvent typical distribution controls, it may undermine the medical system's gatekeeping role and directly enable unhealthy self-medication.
Ensuring Responsible Implementation
As the capabilities of AI for drug discovery and design continue advancing rapidly, ethical considerations and responsible practices must keep pace. All stakeholders - researchers, companies, governments, and oversight bodies - must be proactive in implementing robust governance frameworks.
On an institutional level, organizations pursuing AI-driven drug development need clear ethical guidelines and review processes. Independent ethics boards should evaluate proposed research programs, ensuring legitimate therapeutic aims while scrutinizing any potential dual-use risks. Measures like routine third-party audits can provide accountability.
Data policies are also critical, with tight controls over access to sensitive datasets related to drug targets, molecular interactions, and generative chemistry models. Techniques like differential privacy, federated learning, and secure enclaves can enable collaborative research while preventing data mishandling. Robust anonymization protocols are needed as well.
For commercial AI platforms and services, stringent vetting of customers and individual researchers is paramount before granting access to powerful generative chemistry capabilities. Biometrics, background checks, and activity monitoring should be employed to validate legitimate use cases and identify potential weaponization attempts early.
Promoting Equitable Access of AI in Pharma
While mitigating dangers, it's also important to foster the positive transformative potential of AI for pharmaceutical innovation and affordable global healthcare access:
Public-private partnerships combining commercial AI platforms with academic medical expertise can help democratize the historically exclusive drug development pipeline. Lowering economic barriers allows more institutions to pursue therapeutic candidates, especially for understudied diseases impacting underserved populations.
AI-accelerated drug repurposing could extend the utility of existing approved compounds, finding new targeted applications while minimizing development costs and risks. Automating this matching of molecules to novel protein targets represents a promising avenue.
For developing nations, remote AI-facilitated molecular design could support local drug production at significantly reduced costs compared to traditional methods. Cloud-based AI platforms provide affordable supercomputing power, while automated synthesis planning democratizes manufacturing expertise.
On the consumer side, AI-powered personal medication assistants and telehealth advisory tools can provide customized treatment plan recommendations while monitoring for potential drug interactions or dosage concerns.
Looking further ahead, AI systems comprehensively modeling entire biological systems and disease processes could even enable personalized drug design tailored to each individual's unique genomics and health data. Such precision medicine remains an aspirational long-term goal requiring continued AI capabilities advancement.
Ready for Artificial Intelligence Drugs
Navigating this pivotal technological transition requires a delicate balancing act of proactive risk mitigation and equitable access promotion. Upholding core biomedical ethics while updating governance paradigms for the AI era. Prioritizing patient safety while democratizing drug development pathways.
With foresight and multi-stakeholder cooperation, we can hopefully maximize AI's positive pharmaceutical disruption while mitigating potential negative consequences. The future of developing AI-designed drugs responsibly is one we must thoughtfully construct - leveraging this powerful tool to uplift our collective human health and flourishing.
AI promises to revolutionize drug discovery - both for good and for ill. With appropriate governance and guardrails, this transformative technology can democratize therapeutic development, streamline processes, and ultimately improve patient outcomes worldwide. But without responsible development, it also risks exacerbating societal issues like addiction and enabling new chemical threats. Navigating this double-edged sword will require foresight and diligence from all stakeholders.